Anyone can do parkour or walk on rubble. The quadruped robot went back to school and learned a lot.
meet AnyThis four-legged, dog-like robot was designed by researchers at the Zurich Institute of Technology in Switzerland, and is expected to be used for search and rescue at construction sites and disaster sites. Currently, ANYmal has been upgraded to perform basic functions. parkour Moving, also known as “free running”. Human parkour enthusiasts are known for their amazing agility and acrobatic feats, and while ANYmal can’t match them, the robots can jump over gaps, climb up and down large obstacles, and duck under obstacles. It is said that he was able to successfully operate the aircraft while crouching down. recent papers Published in Science Robotics magazine.
ETH Zurich team introduces ANYmal’s unique approach to reinforcement learning Back in 2019 and strengthened the proprioception (ability to sense movement, action, and position) 3 years later. Just last year, the team performed a trio The customized ANYmal robot was tested in environments as close to the harsh lunar and Martian terrain as possible. As previously reported, ambulatory robots could assist future rovers, reducing the risk of damage from sharp edges and loss of traction in loose regolith. All robots were equipped with lidar sensors. However, they each specialize in specific functions and are flexible enough to cover each other. If one fails, the other can take over the task.
For example, the main purpose of the Scout model was to survey its surroundings using an RGB camera. The robot also used a separate imager to map areas and objects of interest using filters that pass different regions of the light spectrum. The Scientist model had the advantage of an arm with MIRA (Metrohm Instant Raman Analyzer) and her MICRO (Microscope Imager). MIRA was able to identify chemicals in materials found on surfaces in the demonstration area based on how light scatters, and her MICRO on her wrist imaged them up close. The hybrid was more of a generalist, helping scouts and scientists in measuring scientific targets such as rocks and craters.
While the ANYmal robot and similar legged robots have made progress in recent years, significant challenges remain before they can become as nimble and agile as humans and other animals. “Before the project began, some of my research colleagues believed that legged robots had already reached the limits of their development potential.” Co-author Nikita Rudin said:, a graduate student at ETH Zurich who also does parkour. “But I had a different opinion. In fact, I was convinced that we could do much more with the legged robot mechanism.”
ETH Zurich / Nikita Rudin
Parkour is extremely complex from a robotics perspective, making it an ideal and ambitious task for the Swiss team’s next step in ANYmal’s capabilities. Parkour can involve large obstacles, and robots must “perform dynamic maneuvers at their operational limits while precisely controlling base and limb movements,” the authors write. For ANYmal to be successful, it must be able to sense its environment, adapt to rapid changes, and select viable paths and sequences of actions from its programmed skillset. And all of this must be done in real time using limited onboard computing.
The Swiss team’s overall approach relies on machine learning and model-based control. They divided the task into three interconnected components. One is a recognition module that processes data from onboard cameras and LiDAR to estimate terrain. Locomotion module with a catalog of programmed movements to overcome specific terrain. The Navigation module uses intermediate commands to guide the Movement module in choosing which skills to use to navigate various obstacles and terrain.
For example, Rudin used machine learning to teach ANYmal several new skills through trial and error, such as how to climb over obstacles and figure out how to jump off them. The robot’s camera and artificial neural network can choose the best maneuver based on prior training. Another graduate student, Fabian Yenerten, taught ANYmal how to recognize and negotiate gaps in the debris pile using model-based control. She added machine learning to this, allowing the robot to be more flexible in applying known movement patterns to unexpected situations.
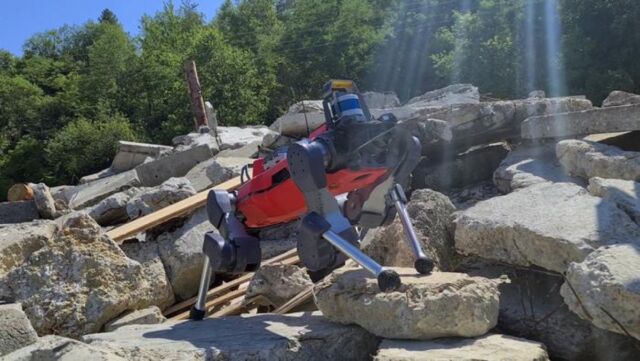
ETH Zurich / Fabian Yenerten
Some of the tasks that ANYmal was able to perform included jumping from one box to a neighboring box up to 1 meter away. To do this, the robot must approach the gap from the side, put its feet as close as possible to the edge, and then, while jumping using three legs, extend its fourth leg and land on the other box. did. You can then move his two legs diagonally and then move the last leg across the gap. This meant ANYmal could recover from any missteps or slips by shifting his weight between his non-jumping legs.
ANYmal was able to not only climb a meter-high box, but also descend from it to reach targets on the ground. You can also crouch and lower your base to reach a goal on the other side of a narrow passageway, adjusting your gait accordingly. The researchers also tested ANYmal’s walking abilities, allowing the robot to successfully traverse stairs, slopes, and random small obstacles.
When it comes to navigating real-world environments, whether it’s a parkour course or the remains of a collapsed building, ANYmal still has its limits. For example, the authors state that they have not yet tested the scalability of their approach to more diverse and less structured scenarios that incorporate a greater variety of obstacles. The robot was tested in only a few selected scenarios. “It remains to be seen how well these different modules generalize to completely new scenarios,” they write. This approach also requires eight neural networks that must be tuned individually, and some networks are interdependent, so changing one means changing and retraining the others. This means that it takes time.
Still, ANYmal “is now able to evolve in complex scenes that require climbing or jumping over large obstacles while choosing a critical path toward a goal location,” the authors write. Therefore, by aiming to match the agility of free runners, we can better understand the limitations of each component in the perception-to-actuation pipeline, work around those limitations, and generally improve the capabilities of robots. We can improve.”
Science Robotics, 2024. DOI: 10.1126/scirobotics.adi7566 (About DOI).
List image by ETH Zurich/Nikita Rudin